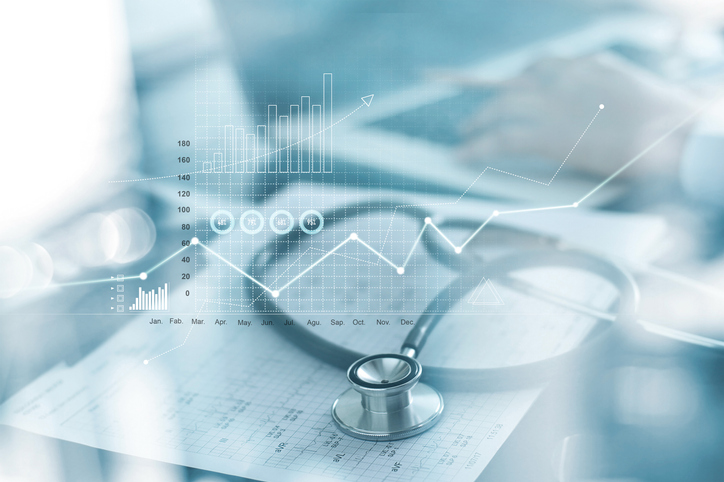
Liberation from memorization: How technology can improve clinical decision-making – MedCity News
Medical education and clinical practice have been based on memorization, and in the case of dermatology—on pattern recognition—for more than a century, and that standard has yet to change.
Something I found frustrating as a medical student and a resident was the amount of clinical data that we needed to consume and process. Physicians and advanced practice providers are expected to commit to memory nearly limitless data, and this expectation begins in school. But, as the healthcare industry becomes increasingly digitized, information is becoming more readily available to us.
We must distill information so that it meets the fast pace of decision-making in healthcare. As an emergency medicine physician, it is not unusual to be managing more than 10 patients at a time—sometimes up to 40 patients at a time—and fielding questions from residents, nursing staff, and others. Trying to remember each patient’s diagnosis, medical history, medication allergy, and other details is impractical.
The challenge lies in finding what piece of the puzzle is clinically relevant out of all that available data. There is only so much information an individual can retain, and this impossible expectation can become overwhelming and can ultimately contribute to burnout.
Burnout not only harms the physician, but it can lead to medical mistakes. A 2018 Coverys report found that the most common root cause of medical professional liability claims was related to diagnosis. These diagnostic errors harm patients and erode their trust in their providers, putting physicians and institutions at financial risk.
We must give the data context so that when a decision is made, the decision-makers—provider, patient or both — feel as though the information is relevant to them. We can achieve this by using technology, like artificial intelligence, in the exam room and beyond.
The goal is not to replace human intelligence but to augment knowledge
Artificial Intelligence (AI) refers to the use of software to simulate human intelligence in programming software. As a result of new algorithms developed over the last five years or so, software can now detect patterns in imagery to identify features of specific diagnoses.
For example, some software can now be used to scan radiologic images to detect changes over time or point to diagnoses. In ophthalmology, an FDA-approved software application can detect diabetic retinopathy with greater sensitivity than an ophthalmologist.
In the field of dermatology, there has been significant focus on machine learning: training software to detect patterns in an image. With machine learning, software can detect patterns in images and indicate the likelihood that a lesion is cancerous, for example. Software could also be used more broadly to analyze an image of a lesion, for instance, and generate a short list of potential diagnoses for a physician to consider.
When considering AI in healthcare, the idea is not to replace the clinician but to support the clinician.
Software could start to see patterns that humans are not seeing, reducing bias and improving outcomes
Humans are biased. We all have these internal shortcuts that we use in our lives, whether we are aware of them or not. Cognitive biases, such as premature closure, anchoring bias, and representative bias, as well as gender and racial biases, all contribute to diagnostic errors and sub-optimal care.
The things we use in our medical education resources and reference materials as well as AI technology in the exam room must be equitable—this is true across all specialties. Consider the evidence showing that women experience more diagnostic errors around myocardial infarction (MI), due to the bias resulting from the mistaken belief that women do not experience MIs at the same rate that men do.
We also know that people with darker skin face worse healthcare outcomes than do individuals with lighter skin. One reason for this disparity is the underrepresentation of dark skin in our educational materials: A recent study found that of the more than 15,000 medical images analyzed across all resources, only 19.5% included dark skin. This lack of representation can affect diagnosis and delay treatment in people of color.
AI can help fill in the knowledge gaps created by these biases. With time and properly developed and vetted software, we could move away from a memory-based system where we create these general statements for people to memorize, to a new world where we augment our thinking based on population datasets. To do that we need to ensure that we are using trusted solutions built by clinicians, developed on diverse datasets, and free from cognitive bias against factors such as race, sex, socioeconomic status, age and more.
The volume of clinical data coming out daily is not sustainable for memorization. Professionals need access to comprehensive and highly reliable knowledge systems as they work. Information technology can ultimately improve care and provide the patient with a better experience in the exam room and at home — not by replacing clinicians, but by supporting them with augmented intelligence.
We need to improve all our medical decisions; to do that, we need to employ equitable, data-driven evidence and tools. By arming clinicians with the right technology, we can foster collaborative relationships with patients, improve experiences and outcomes, and even work toward addressing systemic problems such as unconscious bias and racial disparities of care.
Photo credit: ipopba, Getty Images