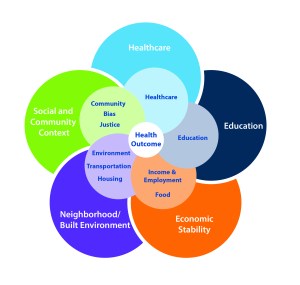
Social Drivers of Cancer Mortality: Part 2 – The Medical Care Blog
Measuring and addressing social drivers of health are important in cancer research. Part 1 of this series, published in March 2022, described three commonly used area-level SDoH indices. None are not able to explain much variation in cancer mortality rates. In this post, I share results from a new model that shows promise.
Methods in brief
Back in January 2021, I wrote about a project that I’ve been lucky enough to lead for the past 3+ years. This award-winning project now has a new name: RTI Rarity. We’ve continued to develop the “artificially intelligent” approach I described last year using random forests (a form of supervised machine learning). We apply a risk adjustment framework and machine learning using more than 150 social drivers of health (SDoH) measures to predict various health outcomes.
Earlier this month, I shared about another RTI Rarity analysis that is using these measures to understand SDoH in places where same-sex couples cluster compared with places where they are absent. These data are incredibly useful in painting a high-resolution picture of social and ecological contexts across the US.
As mentioned in those prior two posts, life expectancy was the main outcome measure of interest in those models. But we can apply the same methods to predicting other outcomes. In this case, we’ve predicted age-adjusted cancer mortality rates per 100k residents. We call the resulting index Local Social Inequity in Cancer (LSI-Ca).
Our random forest models drop variables with low importance, to improve accuracy. So while we start with over 150 predictors, the actual number of predictors retained can vary a lot.
Results in brief
Our pilot model, using 72,770 tracts across all 50 states and DC, included 140 predictors. With a root mean square error of 7.8 and an adjusted R2 of 0.9487, the fit was quite impressive, especially considering our predictor variables consist primarily of social and behavioral measures.
We then moved to modeling each individual state. The variance explained ranged from 61.7% in Nebraska to 100% in 9 states. Interestingly, all 9 models with perfect fit had fewer predictors – between 10 and 30. In all, variance explained exceed 90% in 42 out of 50 states (Figure 1).
Those are the results for our within-state models. Across the entire US, the variance explained in our final model is around 98% (with just 42 variables). Compared to the other composite SDoH indices out there, the LSI-Ca index is quite an improvement.
Measurement challenges
In general, one of the challenges in measuring SDoH is getting data at smaller geographic levels. In the case of cancer mortality rates, the CDC only releases county-level data. The same is true of cancer incidence rates, which (as described in Part 1) account for 31% of the variance in county-level cancer mortality rates.
For the RTI Rarity project, we use the Census tract as our main unit of analysis. Tract boundaries are more stable than ZIP codes (which can change on a monthly basis), and tracts were designed to be relatively homogenous.
Despite the lack of tract-level outcome measure data, we still used the tract as our unit of measure for our cancer models. We simply disaggregated from county level to tract level by assigning the variable’s county-level value to the county’s individual census tracts. This approach, while simple, does have a drawback: maps of the predicted outcome tend to follow county boundaries.
We can deal with this by mapping an alternative model that uses only tract-level measures. The resulting index is not as predictive as our main model (explaining roughly 72% of the variance in cancer mortality rates) but shows more variation by tract.
Putting a bow on it
In Part 1, I concluded by saying:
Three currently available composite measures of SDoH don’t explain much variation in area-level cancer mortality. New approaches are needed to meet this moment.
In this post, I’ve shared the results from our new approach: using random forests to predict cancer mortality rates with SDoH measures. Cancer incidence rates alone explain 31% of cancer mortality rate variance at the tract level. We can improve on that by adding carefully curated SDoH measures across a wide variety of domains. In doing so, we can achieve near-perfect predictions.
This implies that cancer mortality rates are quite sensitive to area-level SDoH — when we measure them in greater detail than generic, factor-based composite indices do. Another implication is that we now can identify tracts in the top decile or top percentile of cancer mortality risk and be pretty sure that we are, indeed, targeting high-risk areas based on SDoH. The final implication is that tailored, disease-specific, comprehensive SDoH indices may be a better fit for some purposes than indices designed to capture SES alone.