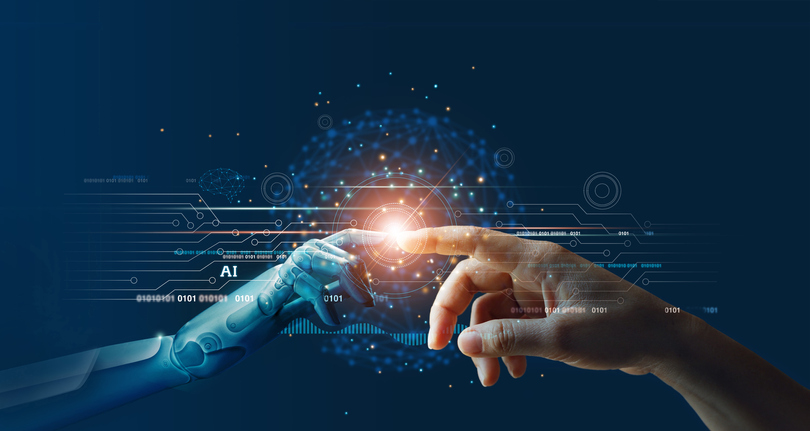
How to evolve artificial intelligence models alongside societal needs – MedCity News
We live in a time when generation-shaping events and emerging technology have converged at a rapid pace – influencing the way our society communicates and interacts with one another. Nowhere is this more evident than with the rise of digital therapeutics in the field of mental health, as new care models and apps are developed for the treatment of physical and behavioral health conditions such as pain, sleep, anxiety and depression.
While it is often presumed that the act of bonding is exclusive to human therapeutic relationships, recent studies have shown that digital therapeutic tools are in fact capable of establishing a comparable therapeutic bond with users. But in the same way that relationships must be nurtured between people, so must the connection between virtual mental health services and their users.
As society becomes more open to and reliant on these tools, it is the responsibility of technology companies, especially those tasked with assisting people’s mental health, to build and maintain artificial intelligence (AI) and machine learning (ML) models that adapt alongside societal needs. But what does it mean to responsibly deploy this technology?
It takes good humans to build AI for good.
We are still a long way off from building AI that can consistently replicate many of the unique traits of long-lasting human interpersonal relationships. Because of this, having an AI generate language algorithmically is fraught with risk, a risk amplified in conversations about health. Evaluation and collaboration between clinicians and technologists is key to identifying where interventions are needed and generating relevant, thoughtful and clinically effective responses.
Ultimately, AI that serves the mental health needs of people can’t be built in isolation. It requires a broad understanding of the human condition, as well as the factors eliciting stress and anxiety in our daily lives. By inserting human oversight throughout the process, developers can build models that reflect people’s lived experiences and the diversity therein, allowing users to more easily develop a bond with a relational agent.
Once a model is deployed, it requires ongoing evaluation.
Regularly evaluating performance and retraining models is critical to ensuring technology keeps up with our evolving world. This process requires a commitment to understanding how individuals interact with digital therapeutics to identify new societal behaviors and adjust responses accordingly. As world events shape the topics users seek to discuss, and emerging vernacular is developed by new generations across social networks, we have the responsibility to follow how society is changing over time and adapt accordingly. This maintenance ensures users feel uniquely understood as individuals throughout their conversations so that therapeutic bonds continue to form.
AI is the gateway to understanding conversation, not the conversation itself.
Even with retraining, AI models must be designed to operate against well-established and validated assumptions, never making unilateral decisions without the user’s explicit buy-in and always leaving the final choice in their hands. While ML can be used to interpret natural language, it should, in effect, mirror reflective listening – with mechanisms to actively confirm the accuracy of what’s being said to users and enable their involvement and collaboration in establishing the path forward.
For example, if a model identifies that a person has an issue with sleep, the conversation flow can be designed to help them confirm that endpoint and reflect. Instead of moving through the conversation, the model can pause to ask, “It sounds like you’re dealing with sleep issues, do I have that right?” If the answer is no, the conversation can move forward in a more informed manner.
Ultimately, each step must be carefully designed to do no harm and support better care. Even if we build great models, without great conversation design, and without preserving human autonomy, we will not enable clinical outcomes or improve mental health.
Never has there been more urgency to address the ethical complexities presented by digital mental health tools. Ultimately, we are in service of improving outcomes for users, and it is our responsibility to consistently evaluate people’s emerging needs and build fail-safes in our systems to ensure users have the autonomy to shape their own journey. With a proactive approach grounded in the authenticity of human relationships, and guided by principles of transparency and preserving self-determination, we can build dynamic digital experiences while staying accountable to our users and ourselves.
Photo: ipopba, Getty Images